Improve AI Model Accuracy
Review real user messages in the Labelling section to associate them with the correct intent. By classifying user messages, your bot's AI model will improve and be enriched with authentic user phrases. Labelling is also used to estimate the bot's accuracy.
Overview
Regular labeling is necessary to progressively improve your chatbot’s understanding. For each intent, you will receive a batch of sentences extracted from real user conversations for labeling.
The user messages selected come from real conversations. Irrelevant messages like test conversations (from the platform), schedulers, and quick replies are excluded by default. Duplicates are also removed to avoid labeling the same sentence multiple times.
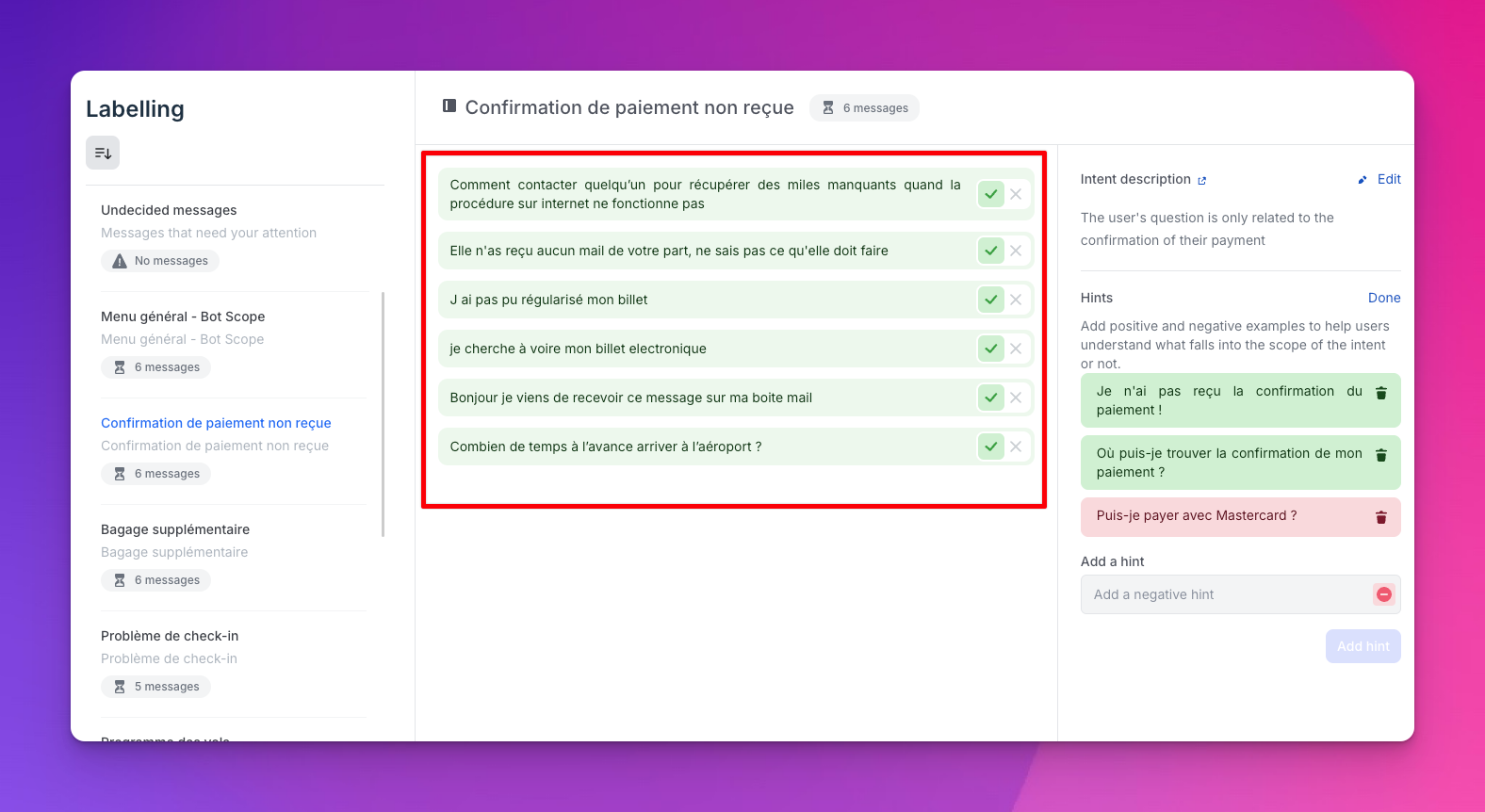
Each message can be labeled in two ways:
- ✅ Confirm the message should trigger the selected intent.
- ❌ Reject that the message belongs to the selected intent. Rejected messages will be sent to the Undecided messages for a final review.
Once all the displayed messages are labeled, you can approve the labelling session. A new set of messages will be displayed until all messages for the selected intent are labeled.
The Intent description and hints help define the scope of the intent more precisely. Both can be edited from the right panel or from the intent page (opened in a new tab by clicking the icon on the right side).
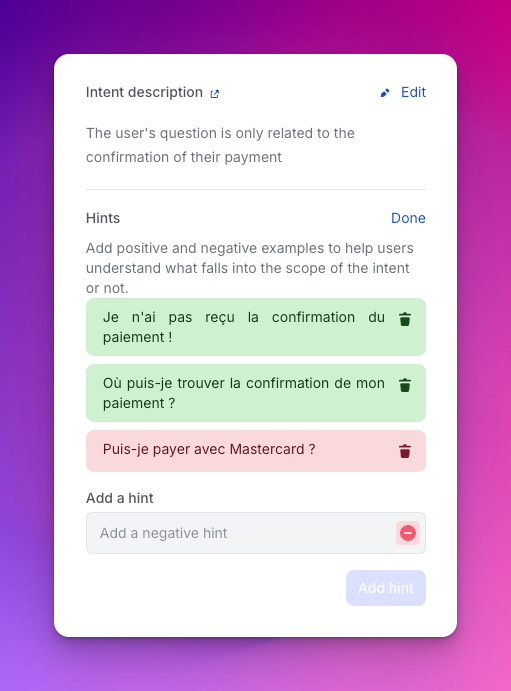
Continue by selecting another intent from the left panel and reviewing all available intents.
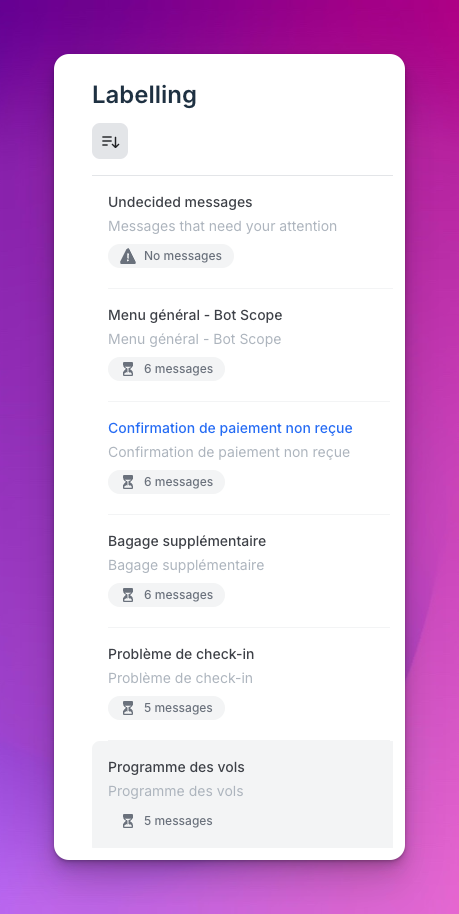
Undecided Messages
If a message is labeled negatively, it is sent directly to the Undecided messages section to pick the correct intent.
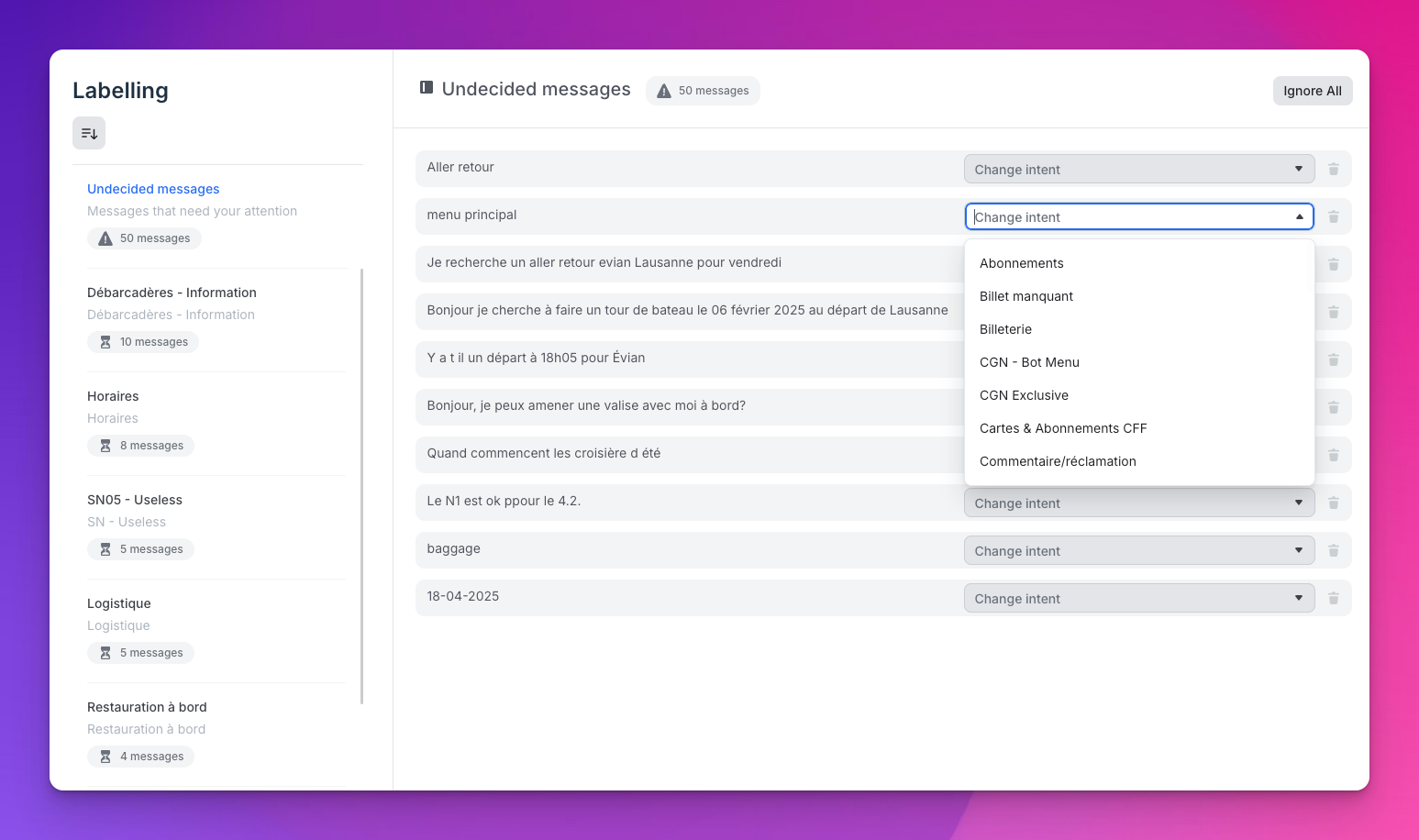
At the top of the intent list, you will see Undecided messages, which gather all rejected sentences from intent batches. An Ignore All button allows you to quickly discard all the Undecided messages if you do not want to consider them.
A Message That Was Ignored Once Won't Be 'Lost' Forever
If the same message is fetched again in a new labeling batch, it will directly go to the Undecided section.
Labelling Batch
Labelling works in batches of messages: a batch consists of 300 messages, randomly selected across all intents of the bot.
Unlock Labelling Feature
If a bot has not yet handled 300 messages, a minimum of 100 messages is required to create the first labelling batch. Test messages (sent from the platform), schedulers, referrals, and quick reply payloads are filtered out of the selection as they are not relevant to label. Messages that are labeled come directly from real conversations between users and your chatbot.
To complete a labelling batch and receive a new one, you need to label messages for each intent and also label the undecided messages. Once the whole session is approved, you can request a new labelling batch.
Train Your NLU Model After Completing a Batch
A few minutes after completing a labelling batch, you will receive a notification to train the Start User Action again.
Accuracy Rate Calculation
When a batch of messages is labeled:
- 80% of the labeled messages are automatically sent to the appropriate intents as training phrases to enhance the bot's understanding.
- 20% of them go to the validation set, which is used to measure the bot's accuracy
For example:
- You label a batch of 1000 sentences.
- 800 labeled sentences are used for training.
- 200 sentences go to the validation set to calculate the accuracy rate.
The accuracy rate is calculated as follows:
- Take the labeled message from the validation set.
- Evaluate if the labeled message is correctly classified to the intended intent.
- Measure the percentage of messages correctly classified.
The validation set contains a maximum of 1000 sentences. If you exceed this, the oldest ones will be removed.
Updated 2 months ago